Note
Click here to download the full example code
Group Lasso RegularizationΒΆ
This is an example demonstrating Pyglmnet with group lasso regularization, typical in regression problems where it is reasonable to impose penalties to model parameters in a group-wise fashion based on domain knowledge.
# Author: Matthew Antalek <matthew.antalek@northwestern.edu>
# License: MIT
from pyglmnet import GLMCV
from pyglmnet.datasets import fetch_group_lasso_datasets
import matplotlib.pyplot as plt
Group Lasso Example applied to the same dataset found in: ftp://ftp.stat.math.ethz.ch/Manuscripts/buhlmann/lukas-sara-peter.pdf
The task here is to determine which base pairs and positions within a 7-mer sequence are predictive of whether the sequence contains a splice site or not.
Read and preprocess data
df, group_idxs = fetch_group_lasso_datasets()
print(df.head())
Out:
...0%, 0 MB
...8%, 0 MB
...16%, 0 MB
...24%, 0 MB
...32%, 0 MB
...40%, 0 MB
...48%, 0 MB
...56%, 0 MB
...64%, 0 MB
...73%, 0 MB
...81%, 0 MB
...89%, 0 MB
...97%, 0 MB
...100%, 0 MB
...0%, 0 MB
...0%, 0 MB
...0%, 0 MB
...1%, 0 MB
...1%, 0 MB
...1%, 0 MB
...2%, 0 MB
...2%, 0 MB
...3%, 0 MB
...3%, 0 MB
...3%, 0 MB
...4%, 0 MB
...4%, 0 MB
...4%, 0 MB
...5%, 0 MB
...5%, 0 MB
...6%, 0 MB
...6%, 0 MB
...6%, 0 MB
...7%, 0 MB
...7%, 0 MB
...7%, 0 MB
...8%, 0 MB
...8%, 0 MB
...9%, 0 MB
...9%, 0 MB
...9%, 0 MB
...10%, 0 MB
...10%, 0 MB
...11%, 0 MB
...11%, 0 MB
...11%, 0 MB
...12%, 0 MB
...12%, 0 MB
...12%, 0 MB
...13%, 0 MB
...13%, 0 MB
...14%, 0 MB
...14%, 0 MB
...14%, 0 MB
...15%, 0 MB
...15%, 0 MB
...15%, 0 MB
...16%, 0 MB
...16%, 0 MB
...17%, 0 MB
...17%, 0 MB
...17%, 0 MB
...18%, 0 MB
...18%, 0 MB
...19%, 0 MB
...19%, 0 MB
...19%, 0 MB
...20%, 0 MB
...20%, 0 MB
...20%, 0 MB
...21%, 0 MB
...21%, 0 MB
...22%, 0 MB
...22%, 0 MB
...22%, 0 MB
...23%, 0 MB
...23%, 0 MB
...23%, 0 MB
...24%, 0 MB
...24%, 0 MB
...25%, 0 MB
...25%, 0 MB
...25%, 0 MB
...26%, 0 MB
...26%, 0 MB
...27%, 0 MB
...27%, 0 MB
...27%, 0 MB
...28%, 0 MB
...28%, 0 MB
...28%, 0 MB
...29%, 0 MB
...29%, 0 MB
...30%, 0 MB
...30%, 0 MB
...30%, 0 MB
...31%, 0 MB
...31%, 0 MB
...31%, 0 MB
...32%, 0 MB
...32%, 0 MB
...33%, 0 MB
...33%, 0 MB
...33%, 0 MB
...34%, 0 MB
...34%, 0 MB
...35%, 0 MB
...35%, 0 MB
...35%, 0 MB
...36%, 0 MB
...36%, 0 MB
...36%, 0 MB
...37%, 0 MB
...37%, 0 MB
...38%, 0 MB
...38%, 0 MB
...38%, 0 MB
...39%, 0 MB
...39%, 0 MB
...39%, 0 MB
...40%, 0 MB
...40%, 0 MB
...41%, 0 MB
...41%, 0 MB
...41%, 0 MB
...42%, 0 MB
...42%, 0 MB
...42%, 0 MB
...43%, 0 MB
...43%, 0 MB
...44%, 0 MB
...44%, 0 MB
...44%, 0 MB
...45%, 0 MB
...45%, 0 MB
...46%, 0 MB
...46%, 0 MB
...46%, 0 MB
...47%, 0 MB
...47%, 0 MB
...47%, 0 MB
...48%, 0 MB
...48%, 1 MB
...49%, 1 MB
...49%, 1 MB
...49%, 1 MB
...50%, 1 MB
...50%, 1 MB
...50%, 1 MB
...51%, 1 MB
...51%, 1 MB
...52%, 1 MB
...52%, 1 MB
...52%, 1 MB
...53%, 1 MB
...53%, 1 MB
...54%, 1 MB
...54%, 1 MB
...54%, 1 MB
...55%, 1 MB
...55%, 1 MB
...55%, 1 MB
...56%, 1 MB
...56%, 1 MB
...57%, 1 MB
...57%, 1 MB
...57%, 1 MB
...58%, 1 MB
...58%, 1 MB
...58%, 1 MB
...59%, 1 MB
...59%, 1 MB
...60%, 1 MB
...60%, 1 MB
...60%, 1 MB
...61%, 1 MB
...61%, 1 MB
...62%, 1 MB
...62%, 1 MB
...62%, 1 MB
...63%, 1 MB
...63%, 1 MB
...63%, 1 MB
...64%, 1 MB
...64%, 1 MB
...65%, 1 MB
...65%, 1 MB
...65%, 1 MB
...66%, 1 MB
...66%, 1 MB
...66%, 1 MB
...67%, 1 MB
...67%, 1 MB
...68%, 1 MB
...68%, 1 MB
...68%, 1 MB
...69%, 1 MB
...69%, 1 MB
...70%, 1 MB
...70%, 1 MB
...70%, 1 MB
...71%, 1 MB
...71%, 1 MB
...71%, 1 MB
...72%, 1 MB
...72%, 1 MB
...73%, 1 MB
...73%, 1 MB
...73%, 1 MB
...74%, 1 MB
...74%, 1 MB
...74%, 1 MB
...75%, 1 MB
...75%, 1 MB
...76%, 1 MB
...76%, 1 MB
...76%, 1 MB
...77%, 1 MB
...77%, 1 MB
...77%, 1 MB
...78%, 1 MB
...78%, 1 MB
...79%, 1 MB
...79%, 1 MB
...79%, 1 MB
...80%, 1 MB
...80%, 1 MB
...81%, 1 MB
...81%, 1 MB
...81%, 1 MB
...82%, 1 MB
...82%, 1 MB
...82%, 1 MB
...83%, 1 MB
...83%, 1 MB
...84%, 1 MB
...84%, 1 MB
...84%, 1 MB
...85%, 1 MB
...85%, 1 MB
...85%, 1 MB
...86%, 1 MB
...86%, 1 MB
...87%, 1 MB
...87%, 1 MB
...87%, 1 MB
...88%, 1 MB
...88%, 1 MB
...89%, 1 MB
...89%, 1 MB
...89%, 1 MB
...90%, 1 MB
...90%, 1 MB
...90%, 1 MB
...91%, 1 MB
...91%, 1 MB
...92%, 1 MB
...92%, 1 MB
...92%, 1 MB
...93%, 1 MB
...93%, 1 MB
...93%, 1 MB
...94%, 1 MB
...94%, 1 MB
...95%, 1 MB
...95%, 1 MB
...95%, 1 MB
...96%, 1 MB
...96%, 1 MB
...97%, 1 MB
...97%, 2 MB
...97%, 2 MB
...98%, 2 MB
...98%, 2 MB
...98%, 2 MB
...99%, 2 MB
...99%, 2 MB
...100%, 2 MB 0 1 2 3 4 5 6 7 8 9 ... 930 931 932 933 934 935 936 937 938 Label
0 1.0 1.0 0.0 1.0 0.0 1.0 0.0 0.0 0.0 0.0 ... 0.0 0.0 0.0 0.0 0.0 0.0 0.0 0.0 0.0 1.0
1 1.0 0.0 0.0 0.0 1.0 1.0 0.0 0.0 0.0 1.0 ... 0.0 0.0 0.0 0.0 0.0 0.0 0.0 0.0 0.0 1.0
2 1.0 1.0 0.0 1.0 0.0 1.0 0.0 0.0 0.0 0.0 ... 0.0 0.0 0.0 0.0 0.0 0.0 0.0 0.0 0.0 1.0
3 1.0 1.0 1.0 0.0 0.0 1.0 1.0 0.0 0.0 0.0 ... 0.0 0.0 0.0 0.0 0.0 0.0 0.0 0.0 0.0 1.0
4 1.0 0.0 0.0 0.0 1.0 0.0 1.0 1.0 0.0 0.0 ... 0.0 0.0 0.0 0.0 0.0 0.0 0.0 0.0 0.0 1.0
[5 rows x 940 columns]
Set up the training and testing sets
from sklearn.model_selection import train_test_split # noqa
X = df[df.columns.difference(["Label"])].values
y = df.loc[:, "Label"].values
Xtrain, Xtest, ytrain, ytest = \
train_test_split(X, y, test_size=0.2, random_state=42)
Setup the models
# set up the group lasso GLM model
gl_glm = GLMCV(distr="binomial", tol=1e-3,
group=group_idxs, score_metric="pseudo_R2",
alpha=1.0, learning_rate=3, max_iter=100, cv=3, verbose=True)
# set up the lasso model
glm = GLMCV(distr="binomial", tol=1e-3,
score_metric="pseudo_R2",
alpha=1.0, learning_rate=3, max_iter=100, cv=3, verbose=True)
print("gl_glm: ", gl_glm)
print("glm: ", glm)
Out:
gl_glm: <
Distribution | binomial
alpha | 1.00
max_iter | 100.00
lambda: 0.50 to 0.01
>
glm: <
Distribution | binomial
alpha | 1.00
max_iter | 100.00
lambda: 0.50 to 0.01
>
Fit models
gl_glm.fit(Xtrain, ytrain)
glm.fit(Xtrain, ytrain)
Out:
/Users/mainak/Documents/github_repos/pyglmnet/pyglmnet/pyglmnet.py:864: UserWarning: Reached max number of iterations without convergence.
"Reached max number of iterations without convergence.")
Visualize model scores on test set
plt.figure()
plt.semilogx(gl_glm.reg_lambda, gl_glm.scores_, 'go-')
plt.semilogx(glm.reg_lambda, glm.scores_, 'ro--')
plt.legend(['Group Lasso', 'Lasso'], frameon=False,
loc='best')
plt.xlabel('$\lambda$')
plt.ylabel('pseudo-$R^2$')
plt.tick_params(axis='y', right='off')
plt.tick_params(axis='x', top='off')
ax = plt.gca()
ax.spines['top'].set_visible(False)
ax.spines['right'].set_visible(False)
plt.show()
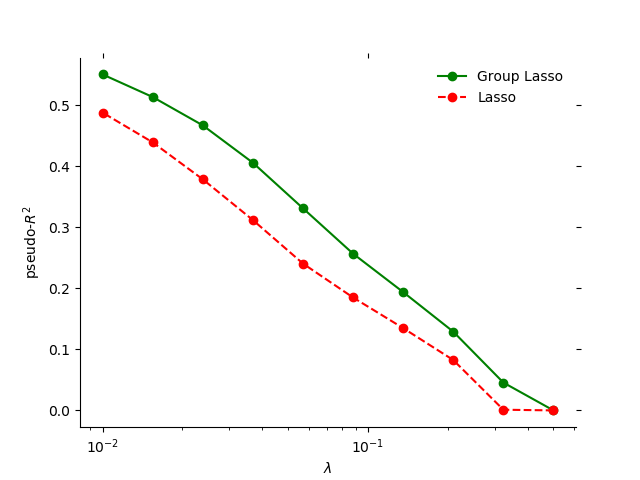
Out:
/Users/mainak/Documents/github_repos/pyglmnet/examples/plot_group_lasso.py:89: UserWarning: Matplotlib is currently using agg, which is a non-GUI backend, so cannot show the figure.
plt.show()
Total running time of the script: ( 3 minutes 21.727 seconds)